How to Move from RPA to Intelligent Automation or Hyperautomation
What is Intelligent Automation (IA)?
To recap, intelligent automation (IA) is the newer variation of robotic process automation (RPA) that leverages cognitive technologies such as machine learning (ML), natural language processing (NLP), and optical character recognition (OCR) among others in place of simpler rule-based automation scripts.
Thanks to a “smarter” technology stack, intelligent automation solutions can handle more complex, multi-step, and data-heavy tasks that require analytical input, rather than just data lookup, entry, or validation.
What is Hyperautomation?
Enterprise hyperautomation is a methodical, need-driven framework for implementing automation across different IT and business functions. Hyperautomation assumes the creation of an orchestrated technological layer for streamlining repetitive processes and implementing fully autonomous workflows.
Hyperautomation tools are not standalone solutions, but rather a collection of different technologies, such as:
- Machine learning (ML) and deep learning (DL)
- Robotic process automation (RPA)
- Intelligent business process management suites (iBPMS)
- Low-code and no-code platforms
- Integration platform as a service (iPaaS)
- Event-driven software architecture
- Adoption of CI/CD and containerized deployments
Check an in-depth comparison of hyperautomation vs. intelligent automation vs. RPA, if you need extra context on each solution.
Many businesses have already experienced the power of RPA – 74% believe in its value proposition, according to an SSON survey. However, 55% of respondents also state that RPA is a useful tactical tool for mostly straightforward processes. As Krishna Edathil, the director of Enterprise Solution Services for the Texas Department of Information Resources (DIR) also noted in the interview:
While RPA adoption has proven to bring significant ROI across many use cases, its prime value comes from topical improvements, rather than sweeping, systematic changes. Hyperautomation emerged as the next evolutionary stage of consolidating localized automation scenarios into a company-wide continuity of automated workflows.
Let’s take the healthcare industry as an example. The front-desk staff uses an RPA tool for organizing patient files and payment details. Clinicians have separate intelligent automation tools that support optical character recognition (OCR) and natural language processing (NLP) for handling clinical notes and organizing patient files in the EHR. However, the administrative staff then need to manually consolidate data between the general patient files and clinical records. This “gap” in automation can be filled with hyperautomation to ensure continuity in execution.
Most companies are now at an active stage of digital transformations – be it transitioning to digital operating models or digital workplace setups. This means that manual business processes are becoming digitized and new digital-native workflows are emerging. Some of them may be redundant or low-effective – and this opens up opportunities to address them with systematic implementation of intelligent automation systems, united into a hyperautonomous setup.
How to Move from RPA to Intelligent Automation and Hyperautomation
The rationale behind adopting any type of process automation is improving the speed and accuracy of its execution.
For process execution to be effective, it needs to follow a pre-defined logic, plus be context-aware. In other words, to complete a task, anyone – a human or algorithm – must understand how to do it and what information is needed.
RPA solutions excel
with “how” but lack the “what” since they were coded to perform a standard task
without any extra deviations. Intelligent automation systems are more
context-aware, but their capabilities strongly rely on data access.
Hyperautomation, in turn, is aimed at building an ecosystem of automated resources provisioning – computing, data, analytics – for supplying the right intel to different intelligent automation systems employed by your organization.
Several conclusions come from the above. First, hyperautomation cannot be implemented without RPA and IA subsystems. Secondly, such solutions require careful planning as you will need to find the optimal paths for connecting a multitude of people, processes, and tools.
App-to-Person Connections for a Company with 1000+ Employees
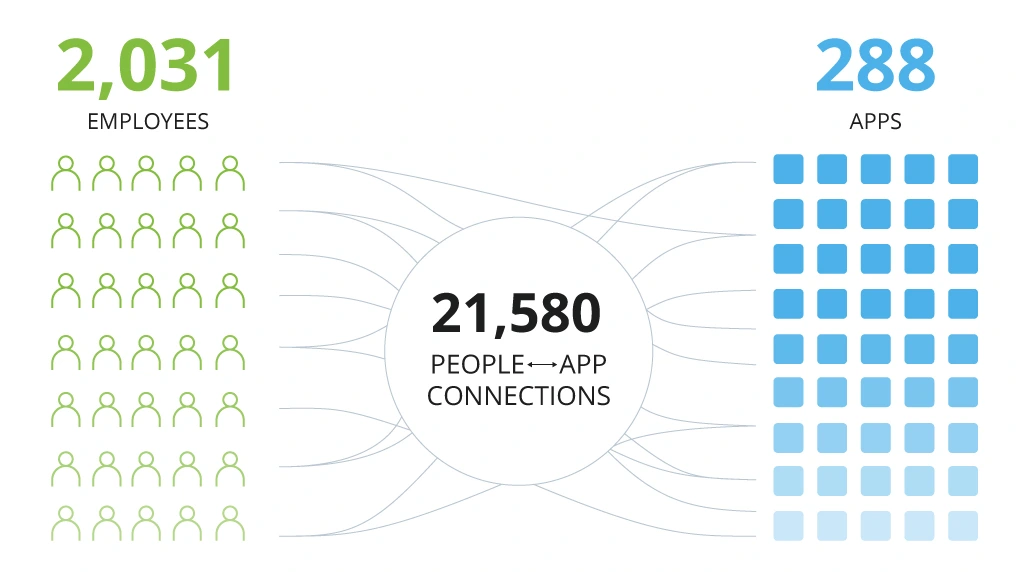
Therefore, to move from RPA to IA and
hyperautomation, you will need to find the optimal ways for connecting
different systems and processes, rather than merely automating one linear task.
To shape your strategy for
hyperautomation adoption, we suggest focusing on the next four vectors:
1. Develop a Strategic Automation Roadmap
To develop a hyperautomation strategy, you need to understand how multiple systems and technologies can be linked together to automate complex processes. As you can easily imagine, multiple combinations are possible – though not every one of them is worth pursuing.
To figure out which processes should be meshed together into one standardized workflow, take into account your business objectives and desired outcomes. The three common reasons for adopting intelligent automation are:
- Operational savings – this can be achieved through increased staff efficiency. Map standard workflows and business processes that can benefit from extra speeds.
- Revenue growth – higher speed and quality of certain customer-facing processes can have a positive impact on your bottom line. Determine which types of automation can help improve CX and/or become part of new product/service offerings.
- Security and compliance – error-prone processes can leave you open to new compliance risks, whereas manual data transfers create extra security issues. The processes automation can minimize the risks of non-compliance or data leaks.
Once you have a preliminary list of processes for automation, analyze them from the performance standpoint. Are these processes subject to frequent changes? As Gladson Baby, Vice President & Intelligent Automation Director, Fifth Third Bank warns:
As already mentioned, the intelligent automation technology is more responsive to changes and allows for on-the-spot “thinking”. Yet, such solutions still require timely access to data and centralized governance. This brings us to the second point.
2. Optimize Your Business Processes
When you have 10 to 20 different ways of executing the same process, any type of automation will fall flat. As part of your roadmap planning, you need to analyze all contenders and locate approaches to the process:
- Standardization to ensure predictable, clear paths to execution.
- Performance optimization to remove redundant steps and/or gaps in logic execution.
- Right-sizing to ensure they indeed fit for producing the desired outcomes.
- Scaling to progressively consolidate the different steps of the business process into one continuous execution flow.
As Gartner notes: “Automation requires organizations to take a new view on key characteristics of processes related to data structure, component delineation and exceptions.”
In other words, you need to understand clearly the nature, components, and data requirements for each process. Then scrub it from all the redundant or excessive elements – and then look for ways to improve the execution with extra decision intelligence (courtesy of big data analytics).
3. Consider Your Data Strategy
When we earlier compared intelligent automation with RPA, we strengthened the importance of data strategy.
RPA solutions are powered by pre-defined business rules. This makes them somewhat limited for business processes with changing contexts. If there is any deviation from the codified standards or missing/incomplete data, the bot will struggle to produce a valid outcome.
Cognitive components of intelligent automation technologies such as ML, DL, NLP make the bots more context-aware. Similar to humans, algorithms can be trained to look up extra data (or patterns within it) to come up with the next best action in an unfamiliar situation.
The above makes access to the high-quality operational data a key requirement to scaling intelligent automation into a hyperautomation ecosystem. To ensure that all tools have unconstrained access to data, you will likely have to re-examine your cloud data architecture – and perhaps implement additional data storage systems such as a data lake or more advanced data fabric.
You can invest in advanced automation tools and have a crisp business process structure. However, without a robust company-wide data governance strategy (and supporting data management infrastructure) you will struggle to scale individual use cases or pursue more complex ones.
4. Map Your Hyperautomation Toolbox
We are bringing up technology selection last as these decisions will be largely dictated by:
- Selected use cases
- Business process maturity
- Data availability
- Prior investments in RPA and other automation solutions
When deciding on new automation technology investments, once again re-assess each step of the business process. At which stages do gaps in auto-execution occur? Why do these happen, e.g., due to the initial process design, lack of data, or lack of technology to perform the automation?
Then consider your approach to filling those gaps. Start with your current toolkit. You may already have several high-performing RPA processes that can be extended with intelligent automation to ensure greater coverage – or be left as-is to co-exist along with such solutions.
Next, analyze other complementary solutions you already have access to. For example, Power Automate is a low-code tool that allows business users to create a range of simple automations and professional developers to rapidly codify more advanced scenarios. The platform also comes with a host of pre-made connectors and sample automation scenarios for other popular business products, such as SAP ERP or Salesforce.
Then consider other proprietary solutions. For instance, UiPath is one of the leaders in intelligent automation solutions. The platform provides access to a wide range of native automation features, as well as great customization capabilities. We recently helped one of our clients move from baseline RPA to a more comprehensive IA setup with UiPath. Post-upgrade, the team scaled to auto-processing over 3,700 tasks per day and saved around 26 FTE/year per one automated process. Find more details in the case study.
Finally, evaluate the necessity for deploying custom IA systems. This option makes sense for highly complex, industrialized processes, such as automatic equipment readings and processing in manufacturing or large-scale computer vision deployments.
To Conclude
Hyperautomation is a
term that frequently emerges among the business chatter, yet its boundaries and
implications are not fully understood. Rather than being a standalone solution,
hyperautomation is a combination of technological means and strategic thinking,
aimed at standardizing, harmonizing, and – only then – streamlining core
business processes within your organization.
In the above sense, hyperautomation is not just the adoption of the new – it is
the optimization of existing technologies, processes, and data exchanges.
Therefore, to succeed in adoption, you need to approach hyperautomation from
both operational and technological perspectives. Decide the main business
outcomes first – then work out optimal pathways to achieving them.
Infopulse provides a spectrum of Intelligent Business services, designed to help businesses accelerate performance, reduce operating costs, and gain access to extra revenue streams. Contact us to receive personalized consultation on the optimal automation path for your company.