7 Uses of Big Data Sources by Industry and Challenges to Tackle Them
Big data and analytics have the potential to completely transform industries in terms of faster and better decision-making, automation, and business processes optimization. Data has never been treated so well and valued so much as it is today. Becoming one of core business assets, it hit unprecedented numbers of success:
- 97% of organizations worldwide already investing in data initiatives
- 60.4% of organizations expect to become data-driven organizations within 2-3 years
- 26.5% report they have already created a data-driven company.
Some industries take a true transformative path and place their big data initiatives at the core of their strategy and mainly, a big data platform. The latter is heavily dependent on big data sources and ways to handle them.
What sources of big data can be used to address specific niche pain points? What are the key challenges of having heterogeneous big data sources and ways to handle them to maximize the business value of your data investments? Dig in to find all the answers in a single article.
What is Big Data?
Big data is a voluminous combination of structured, unstructured, and semi-structured data that organizations collect to address business problems by means of machine learning, predictive modeling, and other advanced analytics technologies.
According to a recent survey, 39.5% of organizations worldwide manage data as a business asset. Within the last two years, the number of organizations that drive innovation with data has risen by 11%. While the systems that process and store big data are becoming a more common component of the companies' data management architectures.
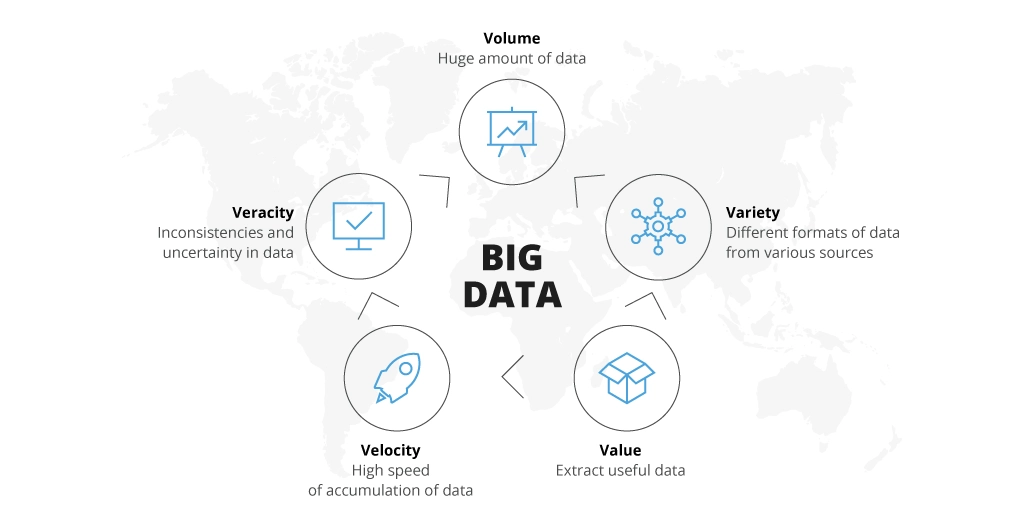
Types and Sources of Big Data
Big data can be classified into various types and sources based on its characteristics. Here are the three common big data types:
- Structured: organized data that follow a predefined format.
- Unstructured: the information that lacks a specific organization and format and requires additional processing before using. This kind of data cannot be easily captured in standard row-column relational databases.
- Semi-structured: such data have some organizational properties (for instance, meta tags) but don't conform to a rigid schema.
At the same time, big data is classified by sources. The three primary data sources include:
- Social: data derived from social media platforms (posts, likes, media file uploads, comments, etc.) and various surveys.
- Machine: data produced by machines, automated systems, or sensors without directly involving humans.
- Transactional: data collected via offline and online transactions at different points of sale.
Here are the examples of big data sources classified by the above parameters:
Big Data Sources by Type
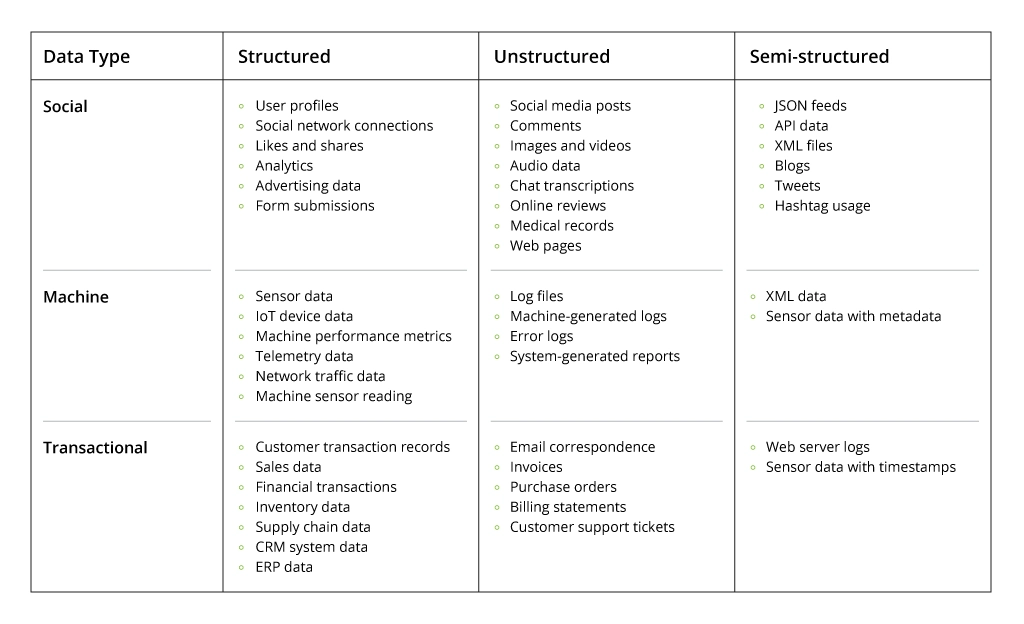
The Use of Big Data Sources by Industry
Big data across industries serves to anticipate customer behaviors, build development strategies, and make more grounded business decisions among other things. The data generated in different industries vary by volume, generation speed, and other parameters. It also defines specific challenges in its processing for a specific industry
Let’s see how different sources of big data are used in various sectors.
Banking and Finances
While the finance industry is not native to the digital landscape, after a long process of technological transformation, banks and other institutions actively embrace innovations. The entire financial service sector was revolutionized with big data analytics. The BFSI-specific sources of big data include:
- The core banking systems
- CRM
- Mobile applications
- Card processing systems
- Client 360 data (credit history, credit score, applied documents, etc.)
- Niche service systems (for instance, loan-specific data).
The value of big data highly depends on how it is collected, stored, and processed. In our project for a global audit consulting company, the Infopulse data engineers implemented a 3 TB Data Warehouse based on SAP HANA and Microsoft Azure-based Data Lake for data scientists to simplify data extraction and processing and accelerate the analytic data reporting by 90 times. Read more about the modern types of big data platforms in our blog post.
Manufacturing & Mining
The report from The IBM Institute for Business Value states the following use of big data sources in industrial manufacturing compared to global use:
Transaction and log data can be used to optimize manufacturing and field operations and address their business goals in terms of new product development. Big data collected by sensors are actively used for anomaly detection which significantly helps reduce wastage and downtime.
The sources of big data used to address specific technical and resource planning actions include data from the ERP systems, IoT sensors, and various data from manufacturing equipment and management systems.
Reducing the number of defects based on enhanced big data analytics helped our client, an international group of steel and mining companies, save up to 300 steel sheets per month and decrease the cost of defects detection.
Agriculture
The global market of agriculture analytics is projected to grow from $1.23 billion in 2019 to $3.21 billion by 2028. Big data is used to optimize farm equipment and resources, manage supply chain issues, improve customer experience, plan farm operations, and facilitate decision-making.
Data sources in agriculture greatly depend on the factors that should be analyzed to understand farming behavior – weather, soil, weeds, crops, and animal production, improving food safety, developing biodiversity, and enhancing decision-making and financing. Based on the area of research, the most popular big data sources for agriculture include:
Often, the data is allocated in disparate systems and lacks visibility for being processed effectively. Such cases require consolidating data from all sources into one single storage. As an example of such solution, Infopulse implemented a complex Cloud Data Warehouse based on Microsoft Azure for a large international agro-industrial group. Our data engineers set up aggregating, processing, and preparing data from different ERP systems. As a result, the time and effort to extract necessary data and generate analytical reports was significantly reduced.
Retail and E-commerce
The global market of big data analytics in retail will grow to $25,560 million by 2028 from only $4.854 million in 2020.
Big Data Impact on Retail and E-commerce:
- Elevated customer experience,
- Enhanced personalization,
- Optimized pricing,
- Increased sales,
- Improved online payment security,
- Industry trends prediction,
- Demand forecast.
Retail companies analyze mostly offline data to address these needs, including merchandising, buyers' actions at retail spaces, and POS-terminal and recipe data.
A case in point: Infopulse implemented a high-precision AI-powered surveillance system that collected information about customer behavior in areas of 2,000+ square meters. It helped optimize the customer flow, lower energy costs, enhance store security, and elevate data-driven marketing and promotion.
E-commerce organizations rely more on analyzing online data – tracking user behavior on the website and mobile application, order data from e-commerce platforms and CRM systems, APIs.
Telecom
Telecom companies face two key challenges – gaining new customers and optimizing ever-growing infrastructure costs. Tapping into all big data sources may help telcos expand their capabilities to manage both by improving the following areas:
- Prevent customer churn: analyzing customer behavior and gathering insights lead to higher customer service and satisfaction.
- Enable personalization: predicting customers’ future needs helps improve product and service offerings and enhance customer retention.
- Optimize pricing: big data analytics help dynamically adjust pricing based on network condition, demand dynamics, and other factors.
- Improve network management: looking into the customer's historical data, telecoms can define the reasons for customers' problems, issues in network connectivity, and predict potential failures in the future. For example, here’s how we helped our client, BICS, enable proactive troubleshooting by speeding up signaling data analysis with the newly implemented data platform based on Apache Hadoop.
The key sources of big data for such insights in telecom are call data records (CDR), CRM, ERP data, and various logs from equipment (including routers).
Transport and Logistics
The transport industry relies heavily on data analytics to optimize resource consumption, reduce operational costs, and optimize routing. IoT-enabled connected devices are actively adopted to track deliveries, while temperature and other sensors are used to control vehicle performance and prevent breakages.
In ten years time, the IoT spending by the logistics industry will reach $114.7 billion from $39.6 billion in 2022.
Moreover, the data from ERP, CRM systems, IoT, sensors, and GPS help analyze the current transport performance and routes and create new benefits for future business growth in terms of optimization. Big data sources are used in transport management systems to optimize routing and reduce expenses effectively. Hence, ensuring supply chain transparency and predictability makes moving cargo safer and more efficiently, expanding the fleet capacity and improving customer satisfaction.
Energy, Oil & Gas
Energy, oil and gas companies experience industry-specific challenges related to the lack of operational processes visibility, logistics complexity, lower performance, and various environmental regulations.
Modern equipment and systems allow tracking enough data to address these challenges, and enterprises actively adopt solutions for data-driven improvements. For instance, we helped a leading German Oil & Gas manufacturer reduce the turbine failure probability by promptly detecting abnormal gas turbine behavior with 93% accuracy.
Key big data sources for energy generation and gas and oil extraction include IoT, equipment sensors, and ERP. B2C energy operators (electricity suppliers) also benefit from processing billing system data, data from counters, consumer mobile applications and web portals, and external APIs.
Key Challenges to Handling Big Data Sources
While there are numerous industry-specific challenges big data helps address, the big data itself imposes specific difficulties when processing it, and they are common for most of the use areas.
Here are the typical challenges organizations face when adopting big data to their needs:
- Unmanageable volume: 43% of decision-makers think their organization's infrastructure cannot manage the growing data demands in the future. Potential solutions include creating a scalable architecture and using management and storage technologies to process effectively the growing data volumes. Cloud solutions based on AWS or Microsoft Azure provide flexible, powerful resources and flexibility to manage any data volumes without expanding the in-house infrastructure.
- Bad data leading to bad results: Quite often, data from the available sources is insufficient for generating meaningful and accurate business insights. Established data governance, access control, and effective process for sorting, cleansing, filtering, and enriching data may help set up adequate data hygiene.
- Multiple data formats: Data in different formats and types can be parsed and re-formatted to derive insights. Sometimes, it requires custom solutions to convert raw data and combine multiple sources into a single storage.
- Numerous sources with integration issues: Integration tools help connect data from various sources (files, apps, databases, etc.) and prepare them for further analysis. Microsoft, SAP, and Oracle offer such solutions. Infopulse developed an innovative big data integration platform for one of the US-based leaders in cloud and big data integration software. The platform features 800+ connectors and integration solutions under PaaS control.
- High cost of data projects and infrastructure can be addressed with effective DevOps and DataOps practices and cost-effective data processing instruments that fit the company's budget.
- Slow time to insight: the time of receiving insights from data until it gets old or irrelevant depends on the big data volumes and your data processing pipeline. To accelerate the process, use modern artificial intelligence technologies and an agile approach to deliver, communicate, and apply your insights faster.
- Security and compliance: security must be a part of the original big data strategy with modern approaches and tools for efficient data protection. The used big data sources must comply with the requirements of your industry and location (GDPR in the EU, HIPAA in the US healthcare, etc.).
Each project for handling big data sources has its peculiarities based on the niche, specific business needs, and the available resources for processing. The technological, operational, and organizational constraints can be effectively managed by choosing the right toolset and future-proof big data infrastructure design. Consulting experts in big data integration also helps address the challenges in advance.
Final Words
Data has become a business asset that generates additional value and tangible benefits to enterprises across industries. The diversity and volumes of available sources of big data allow for solving numerous problems across industries facilitating automation, optimization, cost reduction, and, as a result, progress.
Processing big data imposes specific challenges, mostly related to the following:
- Growing volumes of data
- Disparate data consolidation and pre-processing
- Higher infrastructure costs
Infopulse big data experts are ready to help you get measurable results from your big data investments, addressing the specific needs of your business. We provide Smart Insights services focused on extracting actionable insights from any data.