Moving Towards Real-Time Analytics: All About In-Memory Computing and Self-Service BI
10-minute read
Enter In-Memory Computing
With in-memory computing, the analytical timeframe changes significantly. What is in-memory computing? Traditional business intelligence works by processing data that is held in a relational database on external hardware. In-memory computing, as the name suggests, enables data storage in computer’s RAM. This eliminates the standard I/O processing that is responsible for the slow performance of traditional BI processing.
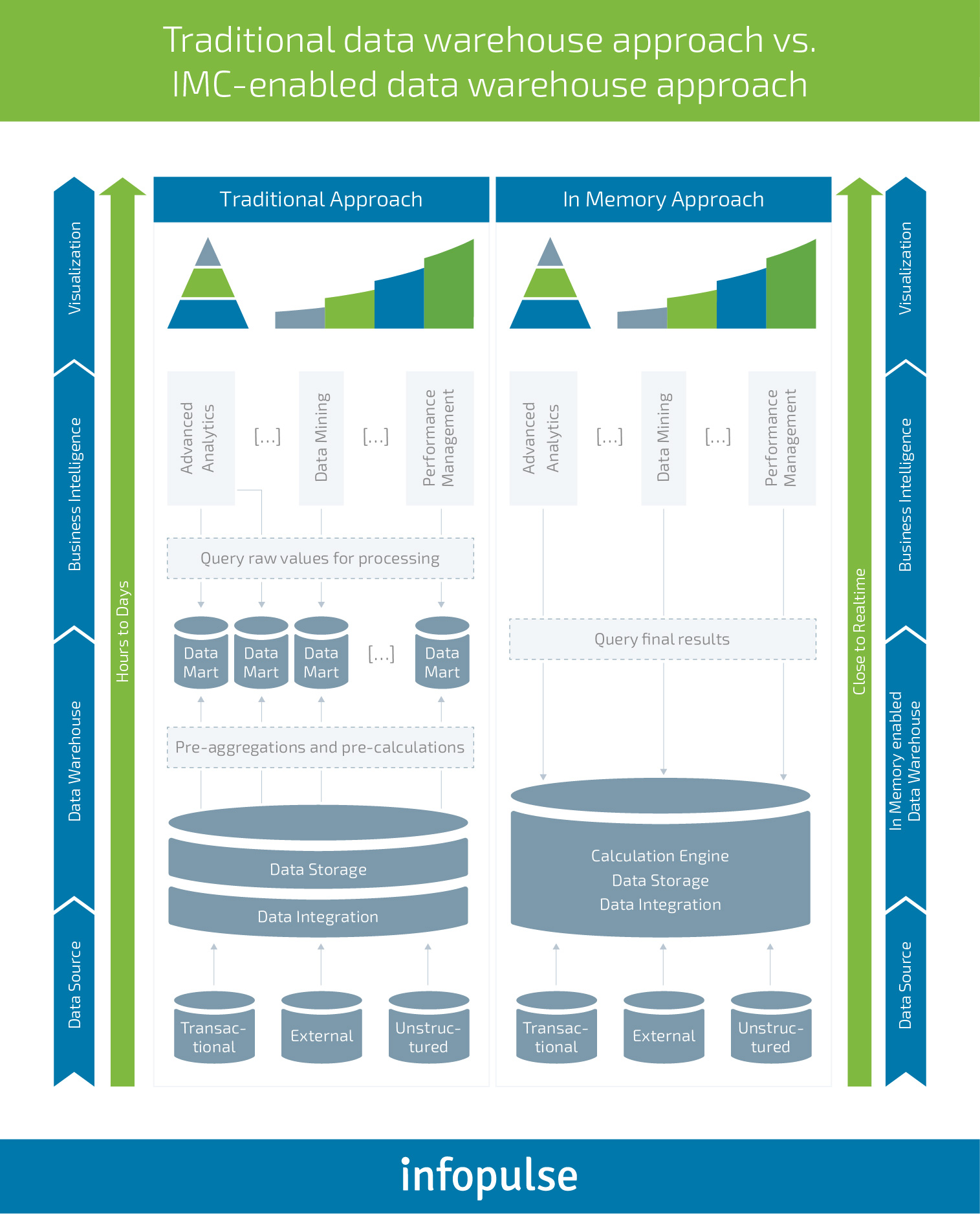
The Business Value of In-Memory Analytics
Data is pouring in at exponential levels, but IT departments and business leaders still struggle to understand how this data can impact their business goals. Traditional analytics tools flop when it comes to analyzing ever-increasing volumes of data, at a speed sufficient to make timely decisions. 37% of executives admit that they need at least one day to access the sources for analytics, per Attivio. In some cases, they require a week or even more time. Additionally, 59% of leaders claim that their legacy data storage systems require too much processing to be in-line with the current business requirements.
Using in-memory analytics and self-service BI, companies can maximize their data access speed and “dig up” deeper insights that can be immediately used for decision making. The infrastructure changes brought in by this approach to analytics eliminate the need to constantly shuffle the data resigning on the disk. As the data is read into memory, you can access it at higher speed. According to Aberdeen Group, companies using in-memory analytics can process 3 times more data at a speed 100 times faster than their competition.
Case in point: SAP HANA has been to create real-time, in-memory analytics for a large trucking operation with fleets in the U.S., Canada, and, most recently the UK. Using data compression, real-time information is stored in RAM, and fleet managers can access this data within minutes. According to Bill Powell, one of the owners of the firm, “There was a sea of information coming in and it could take up to two days to pull it together, which affected our service levels…In-memory HANA means we can answer questions in seconds. Each fleet manager, and even their drivers can generate reports without having to go back and forth with the business.”
There are other benefits of in-memory computing as well:
- First, it creates the ability to better deal with complex event processing. In-memory computing assumes the simplification of the data analytics process due to a reduction in layers. Thus, your team can create simpler, more efficient models that could be tested, modified and deployed in less time. Analytical models can be rapidly adapted to your shifting business needs and new data sources can be plugged in as an additional source of information on-demand.
- In addition to this, in-memory analytics reduces data fragmentation and improves its accuracy. It can help you reduce the timeline for data reconciliation and switch from hindsight to foresight analytics.
- While most databases were initially designed to use maximum memory possible, today’s in-memory processing takes this to a new level. Systems can hold currently running database code as well as active data structures. The persistent database remains in memory. There’s no longer need in any “trips” back to a motherboard.
- Finally, today’s technologies of in-house memory allow for much larger data sets through compression that will compact more data into memory. There are minimal infrastructure constraints when users want to perform analytical tasks and can get immediate results as they come into the system.
Self-Service Business Intelligence: Faster Decision-Making and Other Benefits
As in-memory computing makes data much more accessible, self-service BI is the next logical step to achieve business foresight. What is self-service BI? Read on.
A Self-Service BI Definition
Self-service BI takes data analytics out of the hand of analysts and other IT staff members and puts it into the hands of business users. Users can initiate queries, gather data, create visualizations, analyze it, filter, and sort information on their own. In fact, Gartner predicts that, during 2019, in-house, self-service analytics will surpass the use of data scientists to perform those same tasks.
Before this technology was available, business users would have to send detailed requests for information to team members with experience in data analysis and mining. Then, they would have to wait for the resulting reports to arrive after they were processed. In many cases, if the information wasn’t exactly what they needed, the entire request process would begin again. Only 36% of executives using traditional BI tools are confident that the provided reports and dashboards supply the right data to the right person at the optimal time.
BI self-service tools combined with in-memory computing allow users to get the insights they need on their own with no delay. This, of course, is the most compelling benefit of BI self-service.
Businesses today collect an astonishing amount of data. By implementing self-service business intelligence, users can more easily benefit from that data in ways that improve the customer experience, increase profit, and make business processes more efficient. Here are some other benefits of self-service apps:
- Fast, ready-to-apply insights without the need to engage IT teams.
- “Single version of truth” for all members of your organization. Self-service BI apps tackle information asymmetry within your organization by reducing data silo and giving your company deeper visibility into organization-wide processes.
- On-the-go access to information. BI apps can be accessed from a multitude of devices connected to the network. You team can instantly obtain the insights they need at the moment.
- Low support and training costs.
- Scalability. Your analytics suit “grows” and expands with your data. Most cloud BI solutions will allow you to seamlessly upgrade/downgrade depending on your needs.
- Flexibility. Create custom reports for different groups of users, on-demand.
In-Memory Computing and Self-Service Tools Overview
In-memory computing and self-service BI can help your company gain a new competitive edge by unlocking an access to real-time valuable insights and enabling high-speed analytics of large amounts of data. For this to happen, Infopulse team recommends to take a look at the following four self-service BI tools that have become well-established among business users for real-time analytics and other functions.
1. SAP HANA.
HANA is a SAP in-memory business data platform, combining databases, data processing and application platform capabilities. It’s a prime choice for companies who want run real-time analytics across several environments – cloud, on-premises and hybrid platforms. SAP HANA offers integrated data management experience that increases scalability, while lowering the complexity of your analytics setup. It supports modern applications including IoT, and apps using geospatial or streaming data. Businesses that have already adopted SAP should strongly consider adopting HANA if they have not already done so.
2. Tableau.
One of the many reasons that Tableau business intelligence is so remarkable is that it is one of the very first BI self-service tools on the market. It’s available at relatively low subscription cost for both the desktop and online versions. In fact, it is this low cost that makes the Tableau, a big data grid computing tool, a good choice for businesses that are just dipping their toes into the water of self-service business intelligence.
In January 2018, Tableau introduced Hyper, a new data engine that accelerates query speeds by up to 5X. Data extractions are now 3X faster. Tableau BI tool is an exceptionally stable solution with great support. If any criticism could be levied against it, it would be the fact that the tool was clearly created for power users and those with at least some foundational knowledge of BI. However, with adequate training, users can connect to data sources relatively easily and create useful data visualizations.
3. Qlik.
Qlik BI is another self-service app that uses BI as a means to enable users to create dashboards and analytics apps that they can then use to solve a variety of business problems. Thanks to the associative data indexing engine from QlikView BI tool, users are able to gain valuable data insights and discover relationships in information that is stored amongst a wide variety of data sources.
This self-service analytics tool also provides users with the ability to develop their own custom analytics apps. This can be done with or without coding skills. Considering the time it would normally take to identify a new business requirement, make a development request, then wait for that request to be filled, giving this ability to business users can enable them to respond to changes in business requirements in a much more efficient manner.
Ultimately, QlikView is an in-memory platform that allows IT workers to become less involved in the BI process. The tool has the built-in capability to create data associations automatically and to consolidate data from many sources. This, in turn, provides users with a centralized repository of data that they can use for their reporting needs.
Here is a good example of a project where QlikView was used along with Tableu and SAP HANA.
4. Power BI.
Power BI is an in-memory computing platform created by Microsoft. It has the user-friendly interface that people have come to expect from Microsoft products, along with powerful data analytics and visualization capabilities.
Power BI comes with a total of 74 data connectors with more being added. These connectors allow Power BI to connect to a variety of apps and data sets. These include Salesforce and MailChimp. Users can use Power BI to access data sources individually or analyze data from multiple sources through multiple connections at once. Because of the interface created, this tool is quite easy to use even for users without prior data analysis experience.
Infopulse team has recently helped Ellevio – a leading electricity distributor operator in Sweden – create a new-gen data warehousing and big data analytics solution, which now generates over 70 comprehensive reports accessible via Power BI and other tools. Read more about the developed solution in this case study.
Conclusions
In-memory computing, along with the right BI tools, accomplishes one very important objective. It takes big data and makes it accessible to business users – those who need the information in real time. And if frees up IT departments to focus on other critical business needs.
If you are ready to get on board, schedule a strategic session with Infopulse team. Our professionals will assess your current business needs and help you determine the best self-service BI tool(s) and infrastructure upgrades that should be done to unlock the new level of data analytics.