How Can Manufacturers Take Advantage of IoT, Machine Learning and Big Data?
The next five years, however, are poised to become crucial in terms of growth. The global IoT in manufacturing industry is expected to witness a significant CAGR of 29.4% within the 2017-2024 period. Such exceptional growth can be attributed to several factors:
Increased digitization among enterprise manufacturers and a growing number of SMEs, building their operations using advanced tech stack from day one. The majority of modern ERP systems are already IoT-ready and include gateways to receive information from sensors installed in equipment, making IoT adoption the next logical step towards developing a better line of command.
Rapid advances in machine learning, and deep learning, in particular, enable companies to strategically automate low-value processes and streamline the manufacturing pipeline with the help of AI. New algorithms are capable to operationalize new types of data including audio, video and image cues – the data that is off-limits to the majority of traditional analytics solutions.
IoT interoperability conundrum is being addressed by blockchain. Lack of unified protocols for securely storing and transmitting data has been slowing down IoT adoption across both B2C and B2B verticals. Blockchain – an immutable digital ledger technology – has emerged as a new protocol for seamless and fast data exchanges within large-scale networks of interconnected gadgets. Together, blockchain and IoT can revolutionize supply chain management; improve track and traceability, warranty management and maintenance, repair and overhaul (MRO) processes.
So, what is the Internet of Things role in the future of manufacturing? According to McKinsey data, enterprises identify the next strategic priorities for their in-house IoT projects:
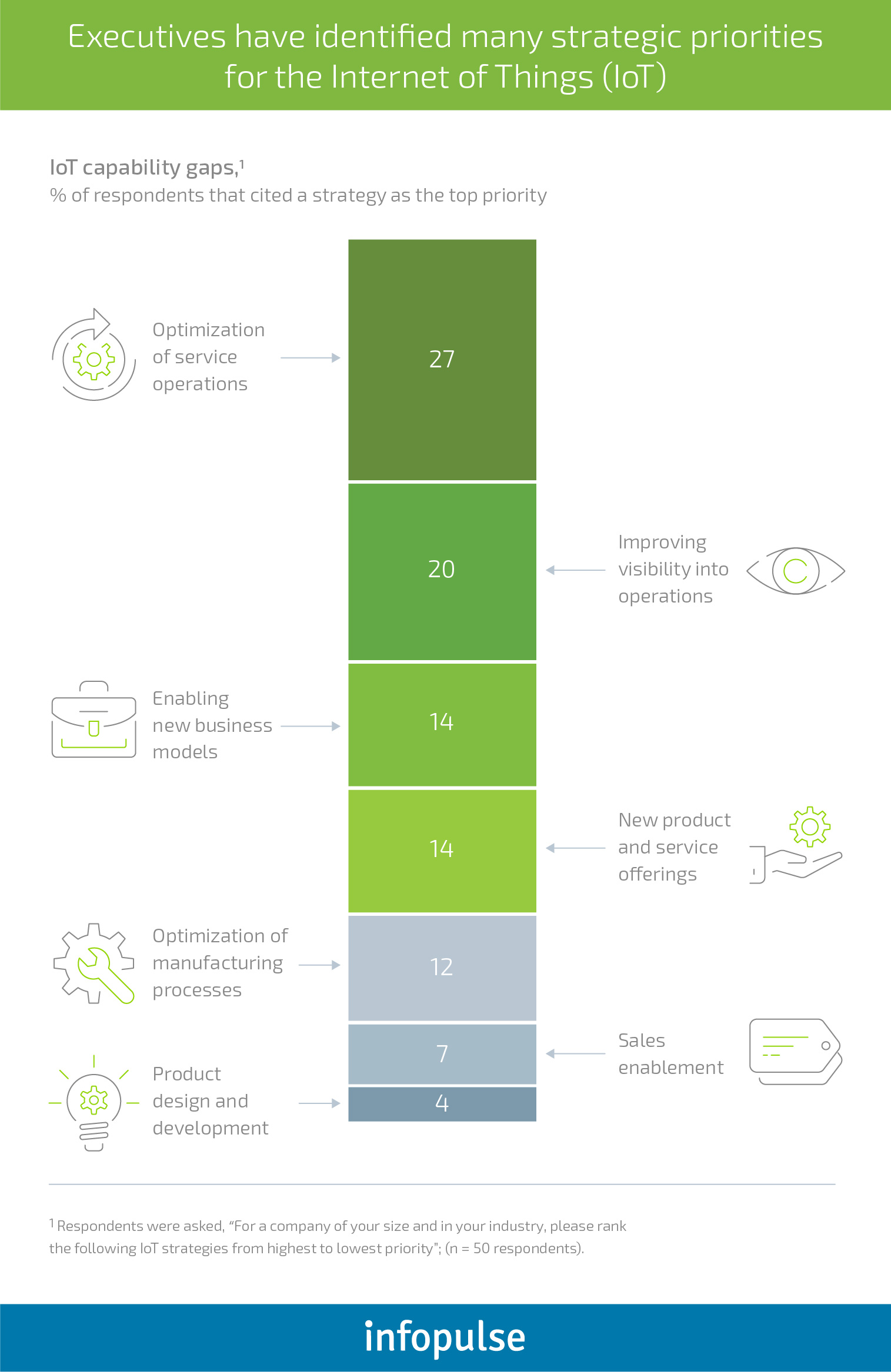
Big Data and Machine Learning: The Two Pillars of IoT-Based Solutions
The Internet of Things and the future of manufacturing are strongly connected to the businesses’ abilities to operationalize Big Data. The majority of enterprises already has certain analytics capabilities to “crunch” proprietary data. The problem, however, is that legacy solutions are no longer fast or effective enough per se. For example, legacy monitoring systems can only locate failures in a few hours before they occur. They come with a limited capacity and visibility into the entire manufacturing process, and can only identify failures at certain verticals. Creating custom engineering algorithms with predictive functionality for each process type is a lengthy and cost-prohibitive endeavor for most business.
Machine learning techniques effectively address these shortcomings. When connected to the proprietary data warehouse, ML-algorithms can provide near-real-time insights from the data at hand. Specifically, the key benefits of machine learning approaches to manufacturing reside in the following areas:
- Greater adaptability to different asset families;
- Easy-to-scale across the entire plant;
- Rapid model development (and self-optimization over time if deep learning is applied);
- Flexible deployment approaches;
- Proprietary historical and infrastructure data gets unclogged and used to maximize effectiveness.
IoT gadgets, powered by ML and connected to Big Data analytics systems, can instantly notify your teams about any abnormalities in the equipment performance such as changes in pressure, temperature, vibration and so on. This enables enterprises to make operational predictions up to 20 times earlier and with greater accuracy when compared to traditional BI tools.
So, let’s take a closer look at the successful Internet of Thing manufacturing examples and use cases.
Industrial IoT Examples and Use Cases, Powered by Machine Learning and Big Data
IoT has the potential to completely revamp the current assembly line setup, just like Ford’s new approach did back in the day. Fully digital factories controlled and managed remotely have already come to existence.
The Internet of Things in manufacturing can enable higher visibility into the production line – from packing to shipment of the final product. Sensors can deliver real-time data that could be further analyzed and translated into better process management and cost optimization suggestions. Additionally, IoT can provide stakeholders with information about lags in production; helping further reduce the waste and unnecessary inventory. For instance, Siemens AG plant – an early example of successful “smart factories” – has roughly 75% of its processes automated, with 1,500 field employees responsible for just operating the software and monitoring the production.
IoT-assisted assembly lines are coming of age. Deloitte describes how such setups can look and function:
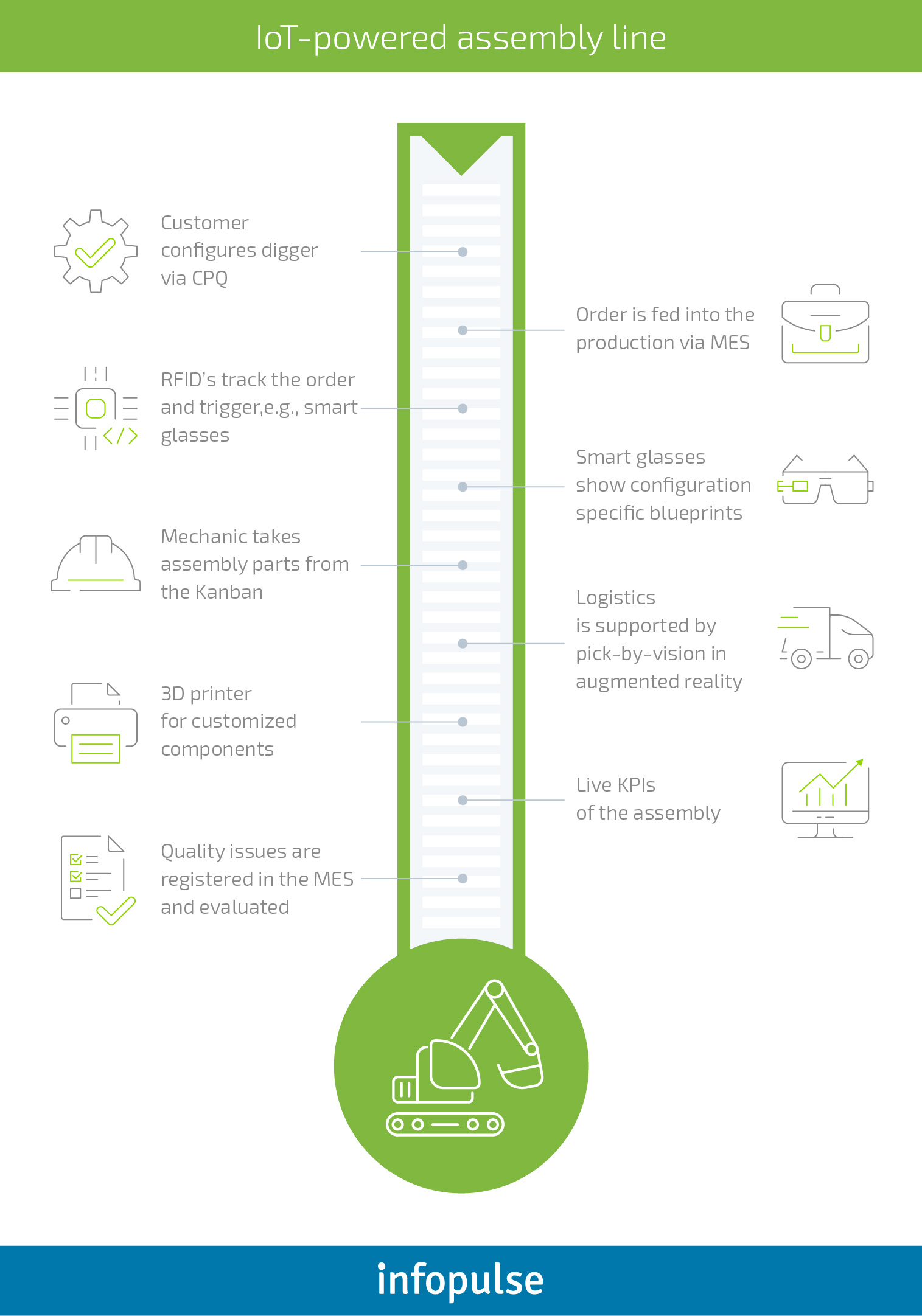
Workers will receive additional support through smart glasses, visualizing the required steps they need to take. Required piecework will be commissioned automatically. Missing or custom parts can be printed in 3D on a per need basis. Each product status remains under constant supervision, ensuring higher standards of quality assurance and increased transparency into all processes. Accumulated data can be leveraged to ramp up operational efficiency even further.
Airbus has already ventured into IoT-assisted production. Employees operating on the factory floor can use tablets or smart glasses to review the task at hand and dispatch into a robotic tool for completion. Such synergy of technology and people has substantially accelerated the manufacturing process as the company execs report.
Digital twinning is another important process, coming to the fore as a result of transitioning towards smarter manufacturing. Companies already have plenty of production data, and one of the ways to use it for better decision-making is to apply it towards creating virtual representations of certain assets that match their “real-word” prototypes.
A digital twin of a product (or one of its components) can be used to simulate the product properties in the early stage of development, without investing in physical pilots and prototypes. Your engineering team can test, validate and optimize various product elements to ensure smooth functioning in advance, identify and eliminate possible gaps. With the help of IoT gadgets, data from simulation models and prototypes can be sent for instant analysis and transformed into actionable product development insights. For example, you can precisely understand how your equipment will operate at extremely low/high temperatures in real-world environments. Digital twinning also enables virtual commissioning of new machinery. Siemens recently partnered with Bozhon – a Chinese manufacturing company – to remotely create an assembly cell with robot arms that was used at a trade show. Such approach allowed the customer to achieve 30% in time savings through accelerated development, delivery and commissioning.
The concept of digital twinning further extends to the production. The “smart” simulation processes can be applied towards visualizing and optimizing every element of the production line:
- Identify possible failures and bottlenecks before the operations begin.
- Reduce the ground time on customizing the setup for mass production.
- Continuously obtain and analyze production data for further manufacturing optimization.
Additional applications of machine learning in manufacturing include the ability to mitigate unplanned downtime, costing industrial manufacturers a hefty $50 billion per year on average. Failed equipment is the root cause for 42% of those outrages.
Predictive maintenance – the ability to predict failure in advance and receive prescriptive maintenance guidance – is one of highest ROI big data use cases in manufacturing. Deloitte estimates that this “intelligent” approach can:
- Cut down the maintenance planning time by 20-50%.
- Increase equipment uptime and availability by 10–20%.
- Reduce overall maintenance costs by 5–10%.
The competitive advantage of ML comes into play here yet again, as AI-algorithms can detect patterns and anomalies on a larger scale than any other analytical solution, driving continuous improvements for businesses. For instance, French power utility EDF Group saved over $1 million on maintenance after deploying a machine learning-driven early warning system on equipment failure.
Another group of machine learning use cases in manufacturing is connected to improved risk management. By pairing IoT devices with ML-algorithms organizations unlock instant access to an array of new insights, enabling better safety management procedures, financial loss optimization and cyber-security mechanisms.
Machine learning can be set responsible for performing regular vulnerability and penetration tests as part of the organization-wide industrial control system security program. This way you can automatically discover gaps in your policies (and infrastructure) and patch them before any criminal tries to exploit them. IoT sensors, placed on key infrastructure, can be tuned to instantly send information whenever any tampering is suspected.
You can also proactively improve on-site employees’ safety and performance by giving each worker a connected wearable device. Fujitsu has recently run a pilot, testing how the new ML-system can capture data to identify threatening heat stress accumulated by factory workers’.
The industry players are also referring to IoT as a way to enable comprehensive quality control at all stages of the product cycle. With the help of big data analytics manufacturing industry can estimate exactly when product issues emerge. Sensors can collect data about the composition and state of raw materials, entering the production facility; temperature and working environment factors and even the impact of transportation on perishable inventory. In-built product sensors can help businesses unlock additional insights about the customer product using patterns. Such knowledge can be then applied to eliminating quality issues or adding enhancements to the newer line of products.
The benefits of IoT further extend towards supply chain management, allowing manufacturers to optimize the shipping process; gain more visibility into how products are handled by the distributors and at what stages the bottlenecks occur. All of that information can be “fed” into ERM, PLM and other proprietary systems for analysis. Capturing such data from suppliers can help you locate the key interdependencies, predict when certain issues may arise and optimize manufacturing cycle times.
The Key Benefits of Modernizing the Production Process with Industrial IoT Solutions
The scope of benefits brought in by the Internet of Things extends across four dimensions:
1. Connectivity.
Traditional manufacturing setups usually present themselves as a collection of loosely-coupled “cogs”, governed by some general rules, often paper-based. By making those “cogs” more intelligent, manufacturers can achieve higher levels of automation, eliminate low-value and unnecessary production steps, and make the entire system function as a cohesive whole. Specifically, Internet of Things automation capabilities lead to the next improvements:
- Data-backed performance measurement and management. Sensors can monitor, record and transmit information related to all steps of the production process – from inspection of incoming materials to shipping. Next, an Internet of Things big data analytics system can combine the internal data with the external one (e.g., supplier specifications, market trends, etc.) and deliver a 360-view of the production system performance. The obtained insights can be applied to continuous optimization of the production pipeline and effective resource planning.
- Improved decision-making and problem-solving. Increased visibility will allow your teams to make weighted decisions about further improvements or changes. Root-cause problems become easier to locate and even mitigate before the occurrence. Predictive analytics tools can warn your personnel well in advance about the possible equipment failures or quality issues. As your system accumulates more data, it becomes capable to predict even more complex problems and offers actionable prevention strategies.
2. Speed.
Encompassing, real-time data collection and analysis make production systems more responsive to changes. Whenever a bottleneck or a deviation occurs, it is instantly flagged for inspection. On-the-ground staff can spend less time on manual optimization and system check-ups as all problems will be diagnosed programmatically, accelerating the entire improvement cycle.
3. Accessibility.
IoT empowers your employees with instant access to the necessary information and tools at the right time. Your teams receive a unified view of the production system and can proactively address any issues. For instance, the analytics interface can present the team leader with current and historical data on a specific process, pinpoint the existing problem and suggest relevant root cause problem-solving tools and best practices.
To capture even more benefits of IoT, you can unlock your system to external entities. Suppliers can track consumption and quality issues in materials, and perform adjustments at their end; auditors can receive a clear outlook into your data and ensure that you are compliant; and equipment manufacturers can review, monitor and control the provided machinery remotely.
4. Anchoring.
Backing up your manufacturing life cycles by Big Data Analytics and IoT means that you can not just create new “best practices”, but also ensure that this new way of doing things is simple. The optimum settings can be just one click away. Your employees should see and experience the clear value of the new system, rather than fret about “how things were easier back in the day”. Smart systems can also increase organization-wide transparency and knowledge flow as all staff receives access to a centralized repository of best practices, ideas and expertise, created by different divisions.
How to Unlock the Power of Industrial IoT in Manufacturing
To align IoT, big data and manufacturing, enterprises should focus on the following four areas:
1. Data warehousing and infrastructure.
Your legacy systems may not suffice for the increased number of data you will need to collect and operationalize. IoT-based projects are typically hosted in the cloud. With on-demand elasticity and pay-per-use pricing, the cloud is a far more attractive candidate for storing Big Data and running large-scale analysis. Consider a robust software framework as well. SAP HANA is a solid choice in this case, as it comes with a host of attractive cloud products and seamless integration with Azure hosting. Accumulating and processing large volumes of data at a rapid pace becomes simple, even when dealing with multiple connected devices.
2. Advanced analytics suit.
Depending on your needs, you may want to either develop a custom algorithm or leverage predictive capabilities that are now pre-furbished into most industrial IoT platforms such as Microsoft Azure IoT, SAP Predictive Maintenance Software, Amazon AWS IoT, IBM Watson IoT and GE Predix.
3. Comprehensive reliability engineering analysis.
Before you can optimize your processes, you will need to establish the baseline metrics. To assess the potential impact and severity of failures, schedule a product reliability analysis, applying different tests to establish how the devices will function under certain conditions within a certain timeframe. Businesses with mature IoT systems can predict issues with equipment, software or service parts months in advance, even before they resurface as a detectable warranty claim.
4. Skilled personnel.
Lack of qualified workers, capable to operate smart plants, is a pressing industry issue. Talent acquisition and on-site training should become the top priority for organizations planning to undergo the transition. While smart systems bring automation at scale, they still need to be supervised and maintained by experienced staff, or otherwise, their value to the production will remain low.
The same goes for off-site expertise, responsible for developing the software back-end of your smart operations. Finding experienced partners will be key to ensure rapid and effective transition towards Industry 4.0.
Infopulse team has successfully helped global manufacturing companies implement industrial IoT solutions and bring transformative changes to their operations. We invite you to schedule a preliminary discussion of your needs with one of our experts.