Financial Fraud Detection Powered by Big Data Analytics
Technological advancements have allowed for the vast and continuous flow of real-time data, which can be used to optimize every aspect of the financial institution: from customer targeting to cybersecurity. On the flip side, a move towards digital banking has brought an array of cybersecurity concerns and fraud risks. Boston Consulting Group reported that financial services companies are 300 times more likely to become a victim of cyberattack compared to other industries.
It has prompted companies dealing with large data sets to implement fraud detection solutions into their risk management strategies. Fraud detection powered by Big Data analytics is used by 75% of respondents who have implemented AI and machine learning in their risk management strategies.
Traditional and Novel Fraud Detection Methods
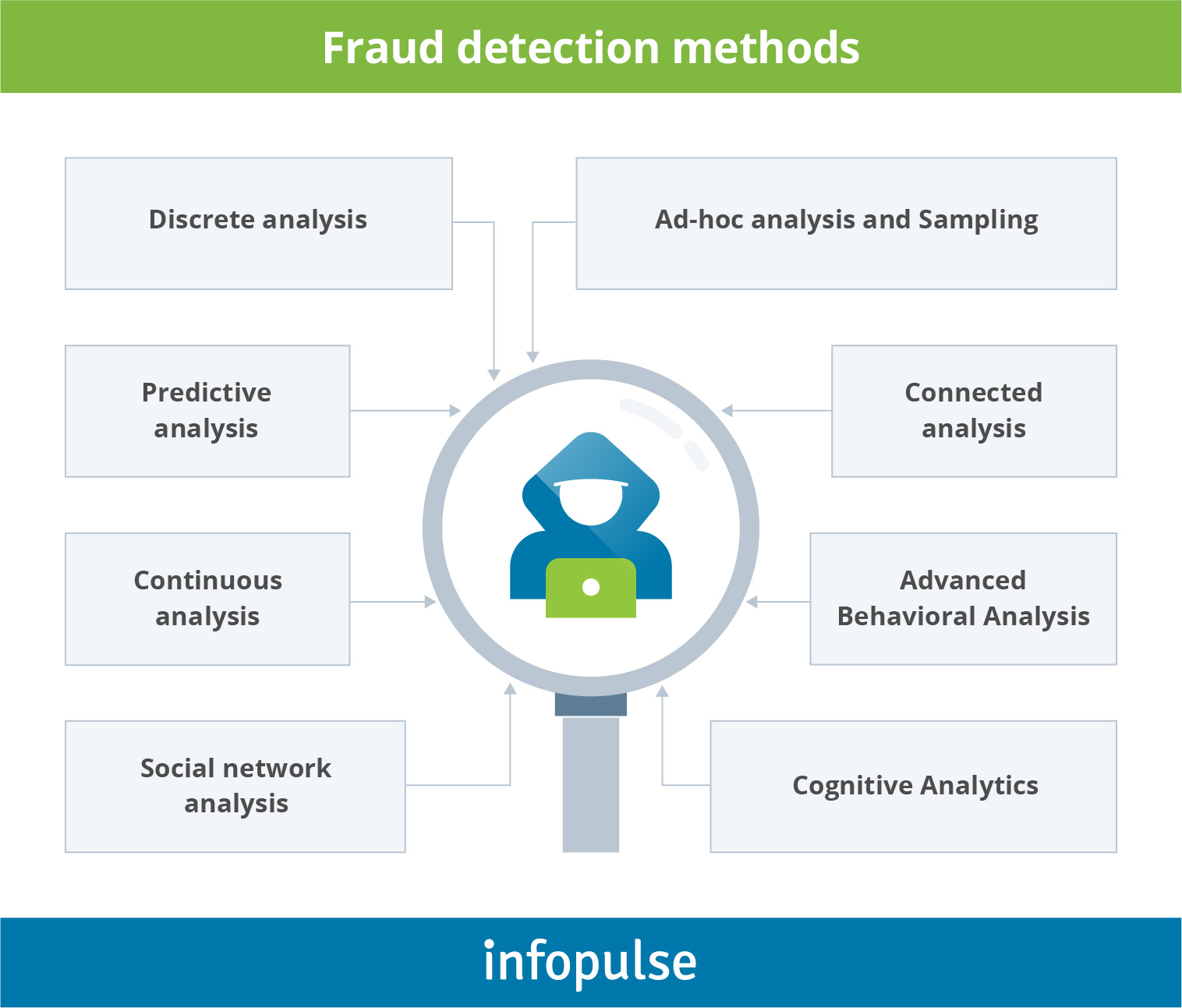
The banking sector has always been on the frontline of fighting financial crimes. However, a global digital transformation and a surge in e-shopping and online banking have made cybersecurity a top priority for financial institutions. Fraud detection is the most efficient way to avoid data breaches that put sensitive customers’ information and the reputation of an organization at risk.
The most common types of financial fraud affecting the banks are identity theft, mortgage frauds, and money laundering. According to a Federal Trade Commission report, credit card fraud is the most popular type of identity theft, which occurred in 41.8% of cases in 2019 (twice as much compared to previous years). An increase in online transactions made wire transfers the most frequently used method for cybercrimes. Such data breaches are time-sensitive, meaning it will cost a consumer only $3 if the fraud is detected on the first day, and it rises to $1,061 if it stays undetected for five months.
Traditional fraud detection methods relied on humans, structured data and discrete analysis. Suspicious actions were detected by a rule-based algorithm and then reviewed manually by investigators. The major drawbacks of such an approach are time, human errors, and inability to identify irregular and unusual patterns of behavior that could result in fraud.
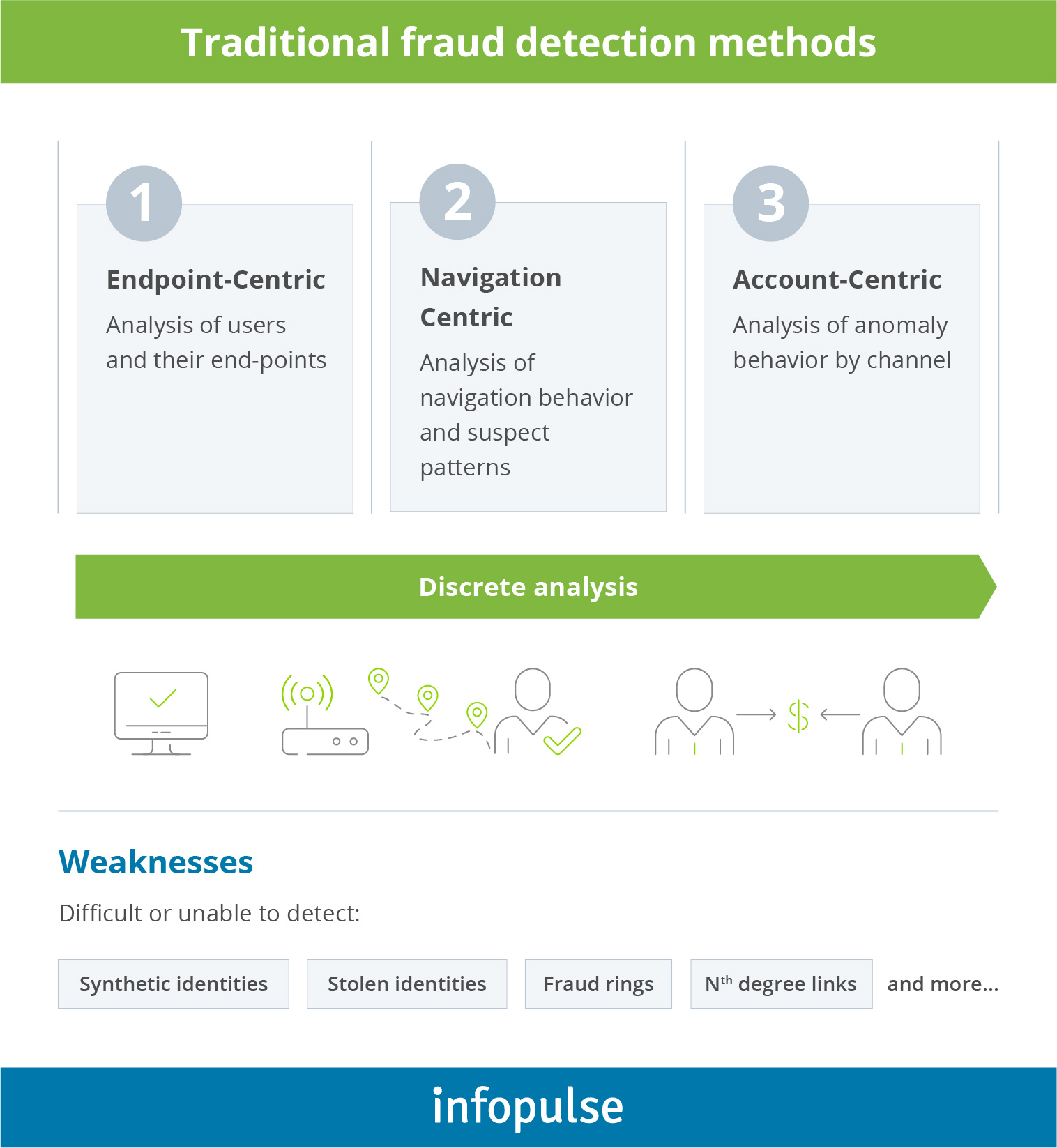
The rule-based algorithm is based solely on rules set manually by an expert, meaning the algorithm would not be able to recognize hidden patterns and predict fraud. Moreover, with an old data warehouse, it is possible to analyze only structured data, like CRM, product silos, and security information, which is stored in an appropriate format.
Novel Fraud Detection Techniques Leveraging Big Data
With digital transformation is gathering pace, customer engagement and interaction are moving online, transforming valuable insights into massive volumes of unstructured real-time data. While it’s a priceless benefit for organizations, it can also become their biggest security crack. AI and machine learning algorithms have allowed for the evolution of fraud detection techniques that leverage Big Data to analyze huge data sets and prevent security breaches. Ad-hoc and predictive analysis represent discrete analysis techniques, when individual actions are assessed.
Ad-hoc analysis and Sampling
Ad-hoc testing is designed to identify specific details about its application area by testing transactions for possible malicious activity. This technique uses a hypothesis as a starting point for checking the transactions for potential fraud. Ad-hoc testing is based on formulas and queries that require manual labor and is time-consuming.
Sampling technique can often complement ad-hoc analysis by providing samples of transactions with fraud risks that can point out some deviations. These methods show good results on small data sets but aren’t that effective on overwhelming data volumes.
Predictive analysis
The main goal of predictive analytics is to create a model that will predict an occurrence of a specific target (suspicious or fraudulent activities). Predictive analysis is accurate if the training set is appropriate for the situation. A significant drawback of this method is that it cannot detect fraud that was not included in the historical data set from which it learned.
Connected analysis
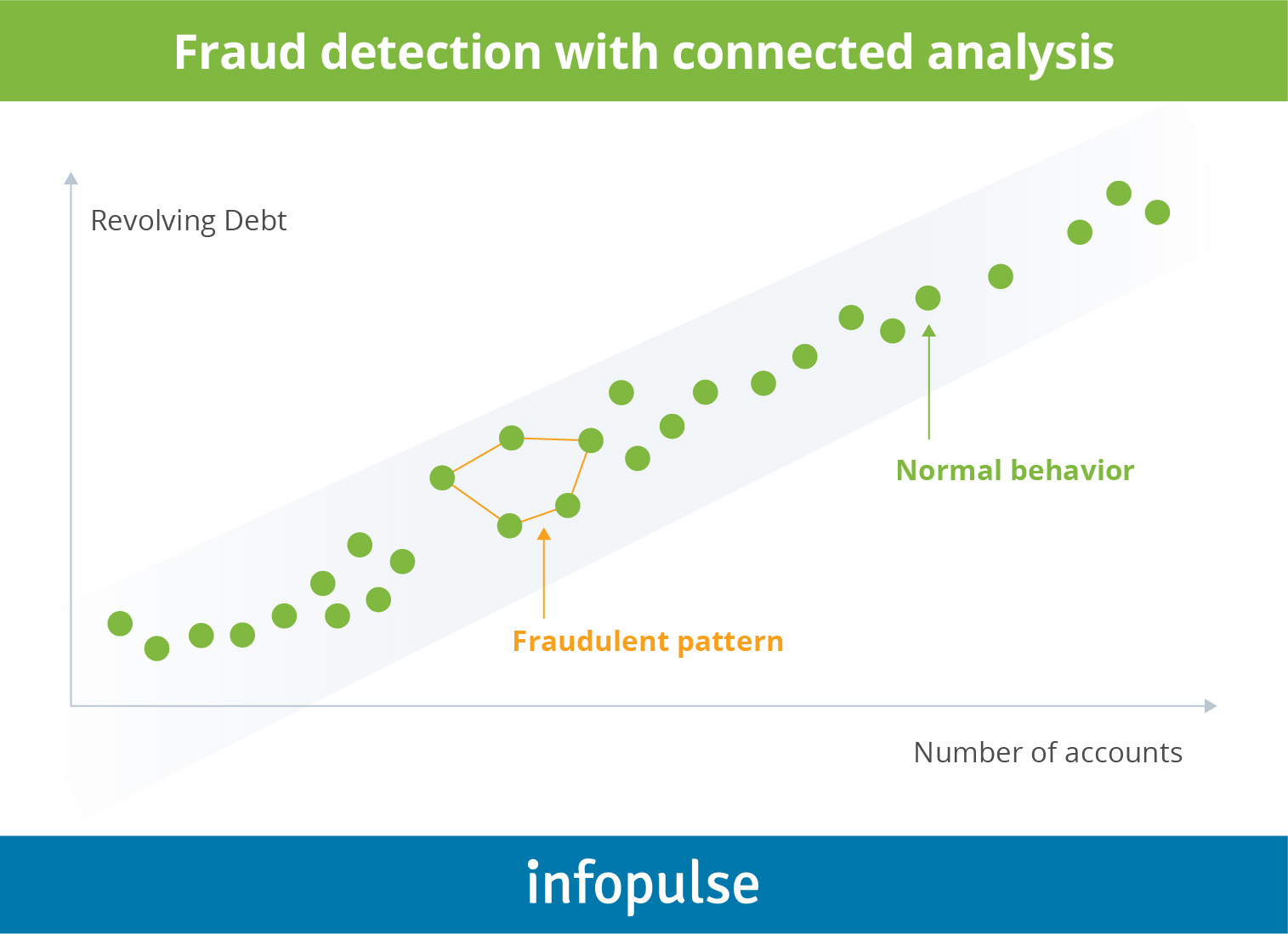
Nowadays, with the increase and diversification of data, a lot of valuable information is hidden in the constant streams of real-time unstructured data, numbers, text, voice, and pictures, including mouse movements and gyro sensor readings, which represent the patterns of customer behavior. When only Google processes 20 petabytes of data each day, the analysis capacity of traditional methods is not enough. That’s where connected data analysis comes in handy. This approach provides a bigger picture of connected behaviors and relationships between subjects that help spot potential fraudulent activities.
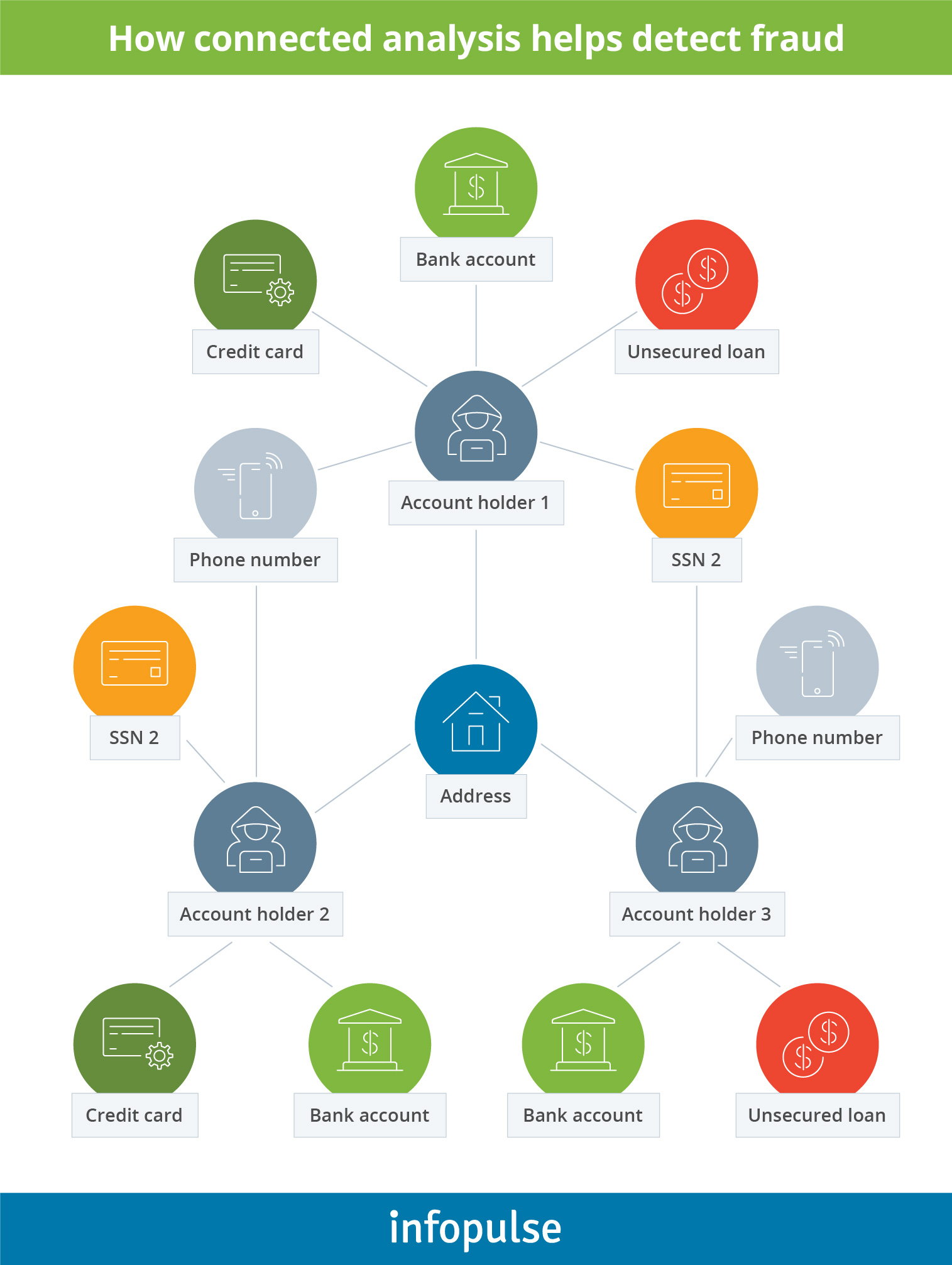
Continuous analysis
This method allows its users to monitor transactions and user activities continuously. An algorithm behind this method can process data and revise its patterns in real-time. The major strength of the continuous analytics is its opportunity to provide new insights based on its findings compared to the more conventional rule-based approach. Consistency is key to fraud detection, with most of the fraud cases running unnoticed for about 18 months.
Social network analysis (SNA)
SNA provides useful insights into large datasets along the network based on the correlation of the analyzed subjects. Social network analysis expands the scope of valuable data by extracting additional value from the subject’s relationships. SNA is a valuable method when it comes to linking many and various data sources for not just fraud detection but prediction as well. While traditional data-driven methods use statistical methodologies, connected analysis techniques rely on relationships between the subjects.
Advanced Behavioral and Cognitive Analytics
The Big Data technology has transformed the data storage with Operational Data Lakes (ODL), which can store both structured and unstructured raw data. Popular Big Data processing platforms like Apache Hadoop, Apache Storm, Google BigQuery, and others provide open-source frameworks, which allow for parallel processing by distributing huge data sets across many servers.
It has opened a novel approach to data processing. Deep analytics techniques represent an evolution from a discrete analysis of structured data towards a connected analysis of unstructured and real-time data. By analyzing the behavioral patterns of each customer (spending, transaction patterns, average balance, geolocation, etc.), the deep analytics system can detect anomalies and red flag a potentially fraudulent activity. It looks for trends and relationships between similar attacks and creates real-time algorithms for spotting suspicious activities. For instance, Danske Bank struggled with cybersecurity for years with a 40% fraud detection rate and 1,200 false positive alarms a day. After implementing deep learning solutions paired with advanced analytics, the bank reduced the false-positive cases by 60% and contributed to the increase of the operating profit.
Those adaptive behavioral and cognitive analytics are efficient at distinguishing between fraud and genuine unusual activities. These algorithms build profiles for customer’s activity type and frequency. When an interaction occurs, the information about the type of device, location, and a number of purchases is evaluated. Those behaviors make a customer portrait that can be used for accurate credit card fraud detection. Biometric data, like mouse movements and clicks, allow machine learning algorithms to examine the very nature of the interaction between users and banking websites, and establish a scenario signaling the possibility of potential malicious activity.
Avoiding Financial Losses with Big Data and Machine Learning
Measuring risk is a top priority for any financial organization. When it comes to millions of sensitive personal files, figuring out a potential threat as quickly as possible becomes a primary objective. According to Javelin Strategy & Research, it takes more than 40 days for a bank just to detect a possible fraudulent activity, which can lead to detrimental financial losses. IBM reports that a typical data breach will cost an organization $3.92 million (12% more compared to 2018 estimates). It demonstrates the importance of predictive analytics adoption to prevent fraud from happening.
Fraud detection solutions powered by Big Data is the best possible way to address global cybersecurity threats. Coupled with machine learning and cloud computing, such solutions facilitate the real-time processing and analysis of massive volumes of streaming data. Case in point: American Express deployed a machine-learning model, which matched customer-related data with algorithms to detect suspicious and fraudulent activity. The data-driven approach has saved the company $2 billion in potential annual incremental fraud incidents.
Moreover, fraud detection solutions make invaluable contribution to the company’s information security and protection from external and internal threats. In the recent years, internal fraud has become more widespread among employees. The internal fraud detection mechanism is similar to that of the credit card type. It is an action-based approach including data collection from telephone conversations, website visits, employee transactions, and other work-related activities for specific employee roles. All these data allow for the creation of behavior patterns for each role, which helps prevent internal fraudulent activities.
Conclusion
Big Data analytics is a powerful and intelligent tool that helps not only to detect security risks and fraudulent activity, but also to prevent them in the first place. After all, data is the most valuable asset of any business. Fraud detection is essential not only in financial terms but regarding customer retention as well. Consumers are hypersensitive when it comes to their security. A majority of respondents admitted that they would rather have their personal photos leaked than have their financial data compromised.
In the modern world, data is not just an IT asset; it is a vital part of digital transformation for the banking and financial industry. The latter requires high level of cybersecurity, which organizations can ensure relying on modern fraud detection solutions powered by Big Data.