How Data Fabric Simplifies Data Management in Hybrid Cloud and Multi-Cloud
To realize value from the growing swells of data, many organizations are sizing up data fabric architecture — a connectivity layer, promising to loosen the rigid boundaries between different cloud environments.
Data Management in Multi-Cloud Environments: Constrained, Not Conquered
An average enterprise today already relies on 2.6 public and 2.7 private clouds to store, process, and exchange data. Moreover, many also have on-premises storage and run certain data-processing applications locally.
Though many business products now have seamless cloud pairing (for example SAP and Microsoft Azure tandem), integrations between public cloud services remain more challenging to establish. A viable multi-cloud strategy requires precise orchestration and cloud resource tagging to ensure that corporate data rests in the right location, under proper security standards, and in a compliant manner.
The second crucial factor is data accessibility — for business users, self-service BI tools, and AI/ML-driven big data analytics solutions. Organizations today have no shortage of options per see:
- Data lakes and data warehouses
- On-premises or virtualized data centers
The challenge lies in establishing effective connectivity between these environments. Firstly, each environment supports data storage and retrieval only in certain formats. Secondly, most proprietary cloud (and on-premises) solutions come with access and tooling usage restrictions. Finally, without a mature data governance policy in place, companies lack knowledge and visibility into where different data assets are stored and what is the shortest path to accessing them.
Given the above, it makes sense why enterprises ranked the following two data integration use cases as the highest priority, according to a 2020 Data Pipelines Market Study:
- Enablement of more effective data cleansing and transformation workflows to improve reporting and analytics dashboards.
- Ad-hoc data querying, discovery, and exploration analysis.
If you are also looking into augmenting your data management, data fabric technology might be the missing link.
The Role of Data Fabric Architecture in Hybrid and Multi-Cloud Environments
As the name implies, data fabric acts as an intelligent “connecting tissue”, melding together disparate data repositories, data pipelines, and data-consuming applications.
In an earlier post, we explained what is data fabric and provided a detailed comparison of a data fabric vs a data lake. If you are new to the subject, please refer to the resource for extra details.
When it comes to distributed multi-cloud or hybrid environments in particular, data fabric resolves the three types of boundaries posed by:
- Data platforms and data architecture
- Clouds and cloud services providers
- Company’s transactional and analytical data operations
Essentially, data fabric acts as a processual layer that uses a combination of human and algorithmic capabilities to run a continuous assessment of all forms of metadata. Then perform mapping, consolidation, and standardization of it. More advanced data fabrics solutions can also execute automatic diagnosing of data inconsistencies and malfunctioning in data integrations and apply targeted automated remedies.
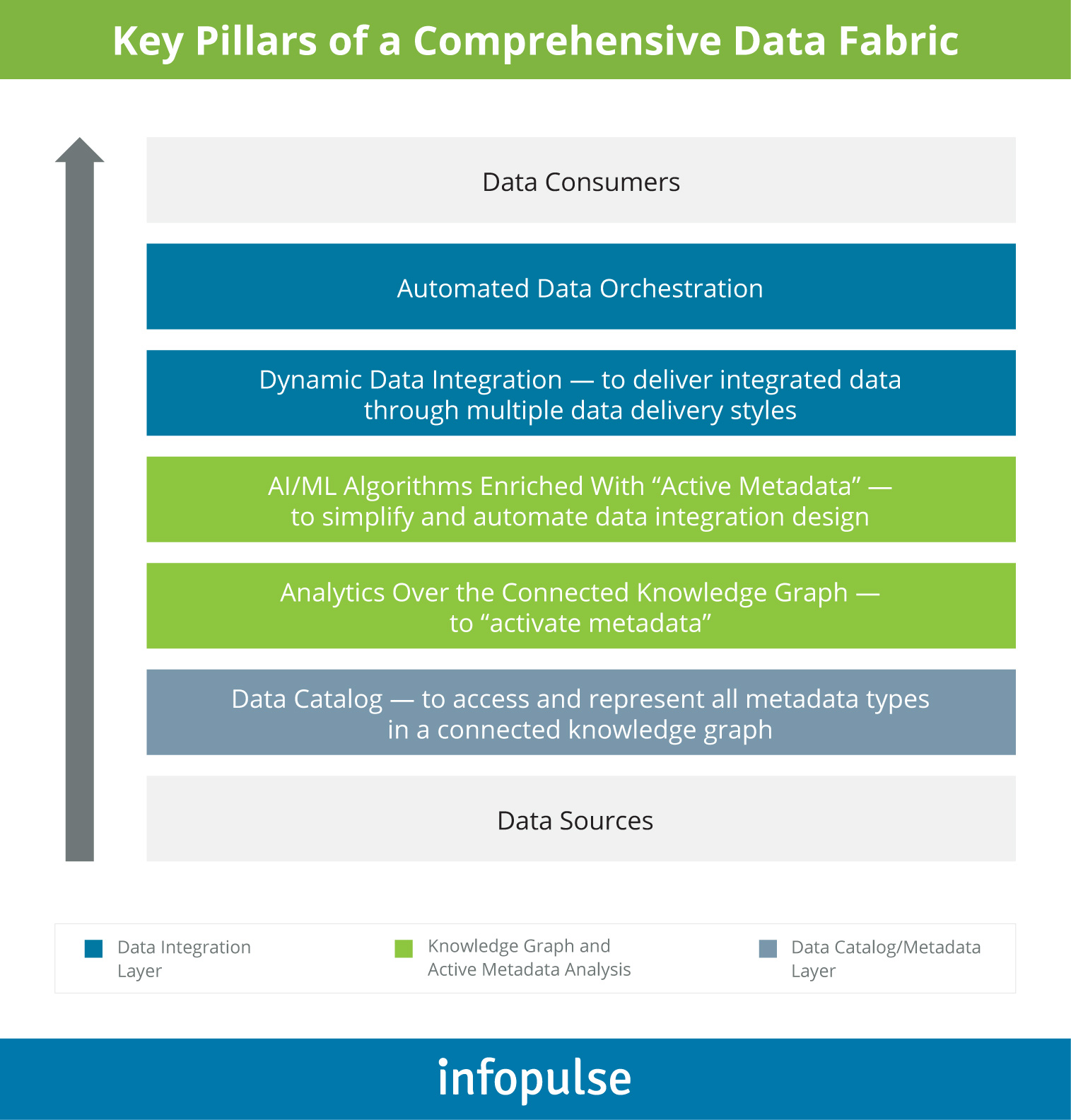
As a result, your organization gains consistent access to consolidated and reusable data from any target location (including hybrid and multi-cloud platforms). Other reported benefits of data fabric architecture include:
- Improved levels of self-service. Data fabric facilitates the creation of detailed knowledge graphs your teams can use to effectively locate, access, and explore different insights.
- Streamlined compliance. Compliance rules and data governance policies can be codified into the fabric with granular precision. Respectively, sensitive data will remain off-limits at all times.
- Automated data integrations. Replace ineffective ETL-based data ingestions with continuous, real-time discovery, and delivery. Data fabric speeds up data processing by several notches and helps establish truly data-driven operations.
A recent study by Forrester, commissioned by IBM for their flagship data fabric product, Cloud Pak, further notes on the “field” gains some of the early adopters experienced:
- 20% reduction in data infrastructure management efforts.
- Between $0.46-$1.2 million in data virtualization benefits — due to improved data governance, security, and accessibility.
- Between $1.2 million to $3.4 million in benefits associated with the implementation of new data science, ML, and AI projects — due to faster model development and deployment, plus a reduction in reliance on legacy analytics tools.
Overall, the underlying idea behind data fabric architecture is to provide organizations with a consolidated, environment-agnostic, singular “control panel” for managing data integrations, governance, security, and compliance across the board. Additionally, data fabric also serves as a staunch alternative to costly and complex data migration projects. Instead of moving the data, you simply establish better access to it.
Principles of Data Fabric Architecture for Multi-Cloud/Hybrid Cloud
Cross-cloud data movement and fast provisioning from and to any target environment is a core issue most enterprises face. To some extent, cloud services providers are to blame.
Cloud interoperability is hard to orchestrate due to by-design constraints each provider implements. There may be connectors available only for data exchanges between certain services. Likewise, the so-called “open source clouds” — infrastructure platforms for developing private clouds or virtualized data centers — also impose certain boundaries when it comes to building direct integrations with other products.
Data fabric provides a “workaround” solution against these constraints. Instead of trying to establish point-to-point integrations, you link the clouds through a separate neutral layer every cloud in your portfolio can interact with.
To achieve the above outcome, the data fabric architecture should be based on the following principles:
- Linear data scalability — the fabric has to accommodate limitless scaling and concurrent access.
- Consistency — it should enforce local data consistency and provide a reference model for implementing the same standards across target environments, without any performance tradeoffs.
- Format-agnostic — ensure equal support of different data types, formats, and access methods.
- Equal metadata distribution — active and passive metadata has to be distributed across all data storing nodes.
- Balanced and resilient infrastructure — data fabric should be logically separated and deployed in a dedicated environment to ensure that heavy loads are isolated and integration of new data sources can be performed with minimal disruption.
- Integrated streaming — to support the rapid processing of data in motion and data at rest, particularly for edge, IoT, and AI applications, while also ensure consistency with the company-wide data management, governance, and security frameworks.
- Multi-master replication — to prevent any losses or inconsistencies, data fabric has to include multi-master replication across multiple locations. Additionally, consider implementing data backup and disaster recovery capabilities.
- Security-by-design — security should be “baked into” the architecture itself, rather than applied to the access method.
How Data Fabric Empowers Value Creation from AI, IoT, Edge Computing, and 5G
Traditionally, applications dictated how data should be organized and stored. Emerging exponential technologies such as AI, IoT, edge computing, and IoT have changed the paradigm of how data is generated when it comes to volume, speed, and formats.
At the same time, the growth of these technologies has increased the demand for:
- Workload volume, speed, and latency
- Cloud data storage and movement
Both of the above factors are crucial for value generation from the above technologies. In these cases, data fabric performs the role of the “enabler”, allowing companies to place analytics atop distributed data sources and apply it towards data on the move.
AI/ML + data fabric. Complex machine learning (ML) and deep learning (DL) models, powering truly innovative products such as autonomous vehicles, wealth management solutions, or predictive supply chain algorithms require significant volumes of training and validation data during development. Post-deployment data processing capabilities only intensify. For instance, Credit Karma captures over 2,600 data attributes per user to assess their credit score and provide personalized financial product recommendations. Data fabric supports rapid processing of such vast volumes of data.
IoT + data fabric. Likewise, industries such as manufacturing, transportation, telecom, and agriculture among others are actively experimenting with IoT and sensing technology use cases. Compact and affordable, the new generation of sensors can effectively collect a wide range of multi-modal data — from pressure and temperature to infrared light frequency and vibrations. Data fabric facilitates timely collection, transmission, standardization, and integration of such “raw” intel for subsequent analysis.
Edge computing + data fabric. The latest generation of IoT devices allows performing preliminary data processing on the device (edge computing). Such a distributed approach to computing reduces latency and reliability issues as data does not have to travel to a connected cloud or on-premises data center at a further location. Modern edge devices and platforms can be programmed to pre-process data locally, and only then dispatch it to the target environment via a well-secured pipeline. Data fabric, in this case, can help standardize metadata management and process governance for a multitude of edge devices your company deploys.
5G + data fabric. In telecom, data fabric can enhance discovery, real-time processing, and distribution of user data to downstream analytics applications. With such a setup, telcos could obtain actionable customer insights and identify new revenue opportunities faster and with higher precision. Moreover, embedding data fabric into 5G network deployments could also enable better network topology and architecture, e.g., self-organizing networks, network slicing, and virtualized network deployments among others.
Time to Apply Agility to Data Management
Unquestionably, cloud computing has revolutionized our way of working. The levels of collaboration, efficiency, and operational speed increased drastically once the internal “corporate” borders of on-premises systems were removed. However, we quickly moved to the next plateau — boundaries between different cloud environments.
Data fabric has come to the fore as a viable solution for removing the current cloud interoperability constraints. Using this technology, you can reduce the time required for locating and operationalizing information, siloed at the farthest technical corner of your organization. For the benefit for any type of user — a system architect, data scientist, or business user looking for a new source for self-service BI tools.